INGE0012
2018-2019
DEEP LEARNING: A REVOLUTION?
Introduction
The first section will review the link between Deep Learning and Data. The history of Deep Learning is closely connected to the history of data storage.
The second section will review the achievements of few scientists who made key contributions to the field. It will then focus on the specific example of games as a driving benchmark for the development of AI.
The third section will analyze the technological impact of Deep Learning in a few key applications, as well as the ethical and societal response to what many consider a major technological revolution of our time.
Computer technology has impacted lives of billions in the last decades. Communication has been made global and accessible, in a fast and reliable way. Access to information is now a given, anywhere, anytime. Computer assisted tasks have been made an everyday encounter, and many jobs require the use of a computer. And innovations brought by computer technology do not seem to have come to an end yet. Automation is getting more and more popular, and the artificial intelligence field are getting more and more attention.
Deep Learning, in particular, has attracted enormous attention recently, in the scientific community, as well as in companies and in the media.
Deep Learning is a recent development of machine learning. Machine learning has a long history made of outburst of results coupled to long period of disinterest, filled with talented scientists. The science of the field is tightly connected to the technology of data and hardware, which are necessary to enable machine learning techniques, and has been conceptualized and studied since the late 50’s. Machine learning received a sudden burst of attention when in 2012 a research team achieve unparalleled results on an Artificial intelligence competition involving image recognition. Attention from the public has grown steadily in the last ten years with new successes of AI in acquiring superhuman performance in games. The latest AI success was the defeat of a professional team of video game players on Starcraft. Those impressive successes have raised great expectation future development of this technology: driver less cars, diseases detection in medicine, supervision of complex systems…
And as all powerful technologies, it also brings its share of ethical problems, and moral dilemmas.
In this blog, we will study the history of deep learning and its impact on technology.
Foreword
This blog is the outcome of the module INGE0012 "Scientific research in engineering and its impact on innovation” on offer at the Université de Liege.
The course is offered to engineering students at the master level. The third edition (2018-2019) was devoted to the impact of deep learning. The two previous editions focused on turbulence (2017-2018) and on cybernetics (2016-2017), respectively.
Students were first assigned to read the recent monograph The Deep Learning Revolution (T. Sejnowski, 2018). The monograph was discussed in class, in addition of a seminar presented by Nicolas Vecoven, a PhD student in deep learning.
Each student engaged individually into bibliographical search with the task of identifying one “source” paper and one “impact” paper highlighting the origin and eventual technological impact of the research in deep learning. Each student prepared a written report on those papers and gave an oral presentation to the rest of the group.
It was then discussed in class how to integrate the raw material into a general blog highlighting the history and the emergence of the deep learning age. The outcome of that discussion was roughly the table of contents of the blog and pointers from each student report to each relevant section.
The final blog was then prepared collectively through a sequence of steps. Each section was first written by a student, then reviewed by another student, and finally revised by the initial writer. Reviews and Revisions were all documented and accessible to all students. Integration tasks were also distributed among students. They included reviewing entire chapters, writing introductory material, selecting figures and writing captions, migrating the final text
to a blog edition, and proof-reading.
At the end of the module, each student compiled an individual report summarising his or her personal contribution to each step of the entire project. Students were also asked to identify and to comment on what they had learned.
For the third time, the response and engagement of the students in this unusual module has been very positive. Many acknowledged that a key outcome of the module had been to realise that science and technology have a history, and that knowing the past is a great asset to shape the future.
R. Sepulchre
Section 1: Let's talk data
The history of data
The word Data is a rather old word that appears the first time around the middle of the 17th Century[1]. Its origin goes back to the Latin word dare that literally means “give”. The word was originally used to describe a fact related to mathematical problems. Later, at the rise of computers, data was defined as a numerical fact collected for future reference. Other new words linked to the data word started appearing like Data-processing, database, and a multitude of other data words. Nowadays, data is defined as facts and statistics collected together for reference or analysis (Oxford dict.).
Data is at the core of any analysis and an important topic that guided most researches and algorithm developments during the past centuries. During these centuries the relationship that people have with data and the diversity of these evolved tremendously.
Nowadays, data is everywhere, everyone makes extensive daily use of them by consulting their mails, by watching videos, or using apps. At the same time new data is also generated for reporting, statistics, forecasting, etc.
Data relationship with people
The relationship that people have with data changed over the years. This evolution is guided by the people’s mentality but also by the technology improvements. Indeed, collecting information about facts and storing them permanently was already the case thousands of years ago. Indeed, the first prehistorical drawings can be seen as the first data the human generated to communicate.
Years later, Egyptians used Papyrus and stones to store and compute data (born of abacus). Then parchments were used to communicate and to store important information but it was costly. During Middle Ages some people, nobles, religious and wealthy people had access to the literature, which can be seen as a form of data.
With the apparition of school and the creation of printing by Gutenberg and the educational system Charlemagne, data increased and allowed a larger fraction of the population to use them and also to generate new data by writing journals.

Figure 1: Historical evolution of data storage
The 20th Century is the period where the relation between data and people changed the most. In particular since the rise of digital -technology field and its democratization, the relation with data has changed dramatically. The possibility to store permanently any type of data opened new opportunities. These opportunities have been understood by companies and companies started to develop products that incorporate the data dimension.

Figure 2: Data is everywhere and used by a multitude of companies, applications, and services.
Some examples can be mentioned such as the digitalization of photographs, the possibility to consume movies, music, and games with CDs. With the improvements of technologies people started to consume and generate numerical data daily without being aware of. Indeed, every day, when most people wake up they check their phones or their laptops to have information about events around the world or consult their mails. Just by doing that, they used data created for them by press companies. In return, they also generate new data that can be seen as feedback that are sent to these same companies. Most of the time people are not aware of that. This data is useful for these companies since they can elaborate forecasting and patterns about people based on this feedback. The relationship that people have with data is now really close. Indeed, data can help people to select a movie, to plan holidays, and, more generally, to make an increasing number of daily decisions.
Data diversity and storage
Computers allow to store data based on a numerical representation. At the beginning, only elementary data was stored and limited in size. Moreover, computers were not accessible to anyone, only big companies and governments were able to access such machines. At that time, computers and data were primarily used to solve specific tasks more focused on achieving complicated calculus.
The things changed with the creation of ARPANET (precursor of internet). At the same time the democratization of the personal computer opened the possibility to people to use this new tool. People fell in love with that tool and it became more and more sophisticated. With this sophistication the possibility to store complex data permanently like texts, images (around 1960s) or sounds became possible. At the same time, due to the interest of people in high-technologies, an explosion of data quantity was observed. To manage this explosion, researches had to find new ways to store data and to reduce the size of storage devices.

Figure 3: Historical evolution of the storage capacity.
The storage field evolved rapidly. Around the 80s 1GB of data was enormous and 1TB practically unimaginable. Nonetheless, 1 GB of data only stores approximaltely 4000 images. It is not that much when compared with today's data. Based on a study [2], each day a household consume more than 400MB. Compared to data of 80s it is enormous and it illustrates the boom of data.
At the same time as the development of storage devices, the computing power also evolved. At the beginning computers were as big as houses and could only manage a restricted number of operations. Most of the time, these operations were not so sophisticated or limited in terms of iterations. But with the democratization of computers and the revenues they generated, companies like IBM, Macintosh and others developed more powerful hardware and reducing thousand times their size.
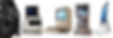
Figure 4: The historical evolution of the computer size
More recently, during the first decade of the 21st Century, a large number of advancements were done in the IT world. Important evolutions included the invention of Smartphones, and above all, the emergence of internet-related companies such as Google, Facebook, Amazon and a multitude of other IT companies. These companies are now investing a large amount of money in machine-learning, in the development of powerful computer and in data storage.
When Global Process Unit (GPU) was created its main purposes was to speed up the creation and manipulation graphical elements to be displayed on screens. But GPUs became a more generalized-device with the Nvidia GeForce 8 Series in 2007. This graphic card version is important since it is the first one that provides a multitude of tools that allow to write code that will use the computing power of highly parallelized graphic cards [3]. It refers as General-purpose computing on graphics processing units or GPGPU. Due to that, graphical cards are highly used in machine learning, statistics, mathematics, imagery… For instance, Nvidia offers diverse tools and libraries specifically created for these domains[4]. The rival of Nvidia, AMD, was a bit late on the optimization of dedicated GPUs for Artificial intelligence and cloud computing but things are changing.
Indeed, more than these two companies started to develop specific devices optimized for machine-learning and deep learning. Google Developed Tensor Process Unit (TPU) dedicated to their data centers and for their framework[5]. On the other hands, Intel developed specific device dedicated to Artificial Intelligence.

Figure 5: Evolution of the deep learning field in relation with hardware evolution.
Data sets over time
Nowadays, data is everywhere with the apparition of IoT Industry 4.0, etc. Each company has measured its importance. Collecting data about people, processes, and facts can help in order to have a better understanding, to bring more revenues to a company, or to solve problems.
Data are also an essential requirement of machine learning. Indeed, to train models and, particularly, Deep Learning algorithms, large data set are required. The creation of such data sets is dependent on the evolution of the data technologies. During the 1960s data sets were rather limited in size due to storage constraints and were composed of numbers and texts. Some years later, around 1980s, more complex data sets appeared due to the increase of storage devices and the sophistication of the computers. In 1995 a data set composed of digital numbers called MNIST was developed[6]. This data set was famous since it was used by LeCun to train its Neural Network but this data set was not huge. Fifteen years later, Fei Fei Li developed a huge data set called ImageNet [7]. This data set is composed of more than 14 million of images separated into 22 000 different categories. This data set is famous because it is the first one with that size and secondly because it became a reference data set used in several machine learning competitions.
After the creation of ImageNet, other data sets were specifically developed for Machine Learning and deep learning models. An example is the data set COCO[8], which is a large-scale object detection, segmentation and captioning data set.
With the improvements made new problems that were too complex to solve in the past century can now be solved. The development of machine-learning models to master games is a good example of this evolution. This topic is discussed in details in Section 2.2. That section retraces the history of games and their complexity from Tic tac Toe to Alpha* algorithms[10,11]. The evolution of the game complexity was influenced by the computer power and data storage. Indeed, for complex games mentioned in that section more than one thousand of CPUs and more than one hundred of GPUs were needed. This amount of power was simply not available 10 years ago.
Open source data
The Open Source movement appeared towards the end of the 20th Century and has had a strong influence on the field of machine learning. Until, the end of 1980s most companies developed proprietary software. To use them these companies charged paid licenses. At the same period some people started to develop the Free software Movement.
The GNU project was born from that movement, followed some years later by the Linux operating System. During the 2000s the Free Software Movement increased in popularity. Other groups were created like Open Source Initiative to promote the open source software. The open source community gave the possibility to people to share their opinions, their views on the development of software, libraries, or tools. With open source software anyone can contribute to a project and the success of a project relies on the community support.
Nowadays about 98% of companies use open source software at their core business since most popular software are in majority open source. It is specially the case for tools related to the machine learning and artificial intelligence. Most libraries and tools are open sources. This is a real benefit since everyone can have access to algorithms implemented. Moreover, it allows people to test thing on their own and perhaps develop models for specific purposes without having an entire knowledge of the theoretical part.
Machines vs Animals
Nature, and in particular the brain, has been a constant source of inspiration for the development of computer technology and machine learning.
Nowadays, deep learning networks have millions of units and billions of weights. This capacity is 10.000 times less than the number of neurons and synapses in the human cerebral cortex. Moreover, the size of the deep learning network units at present can be compared to the brain of a bee [12]. The latter uses a cubic millimeter of tissue with more than a billion of synapses between the neurons.
One measure of the complexity of the synaptic connections in a human brain provided by a rough estimation of its dimension. It has around 100 billion neurons, each connected to around multiple thousand others, amounting to one thousand trillion (1015) synaptic connections. Such a brain consumes around 20 watts to run, while a petascale supercomputer, which is much less powerful than the brain, consumes 5 megawatts. That is, 250.000 times as much power. One reason of this amazing efficiency is the capacity of interconnecting the neurons in three dimensions while transistors on the surface of microchips are interconnected in only two.
The visual cortex has been a dominant source of inspiration for the architecture of artificial neural networks. A retina has one million ganglion cells, and there are 100 million neurons in the primary visual cortex. The representation of a standard object or concept in the cortex needs about a billion of synapses and around one hundred thousand neurons.
Conclusion
This section reviewed the historical evolution of data. The world of digital data is a recent one. It developed in the middle of the 20th Century. Digital data eveolved from simple numbers and texts to more complex elements such as images, sounds, and videos. The development of Machine learning has always been strongly linked to the development of the data technology.
Section 2.1: Intelligent people who made machines intelligent
Introduction: neural networks and deep learning
An artificial neural network is a computational model whose structure is inspired by a simplification of a biological neuronal circuit. This model allows the computer, via an algorithm, to learn by incorporating new data. The field of artificial intelligence that deals with the learning algorithms for artificial neural networks is called deep learning.
Deep learning architectures have been applied in several fields, such as computer vision, language recognition, character recognition, vocal recognition and many others [13].
Deep learning can be defined as a system that uses a class of algorithms that:
-
Use several levels of unit to solve tasks of feature extraction and transformation. Every level uses the output of the previous level as an input;
-
Are based on a non-supervised learning of multiple hierarchical levels of features and data representation. Higher level features are derived from the lower level ones, in order to create a hierarchical representation;
-
Are part of machine learning, namely the widest class of learning algorithms for data representation in artificial learning;
-
Learn different levels of representation which correspond to different levels of abstraction: these levels create a concept hierarchy[13].
How does the learning work in a deep neural network?
The depth of a neural network refers to its number of hidden layers. Traditional neural networks contained 2 or 3 layers, while deep neural networks can contain more than 150 [4]. In Figure1 the structure of an artificial neural network and its hidden layers is shown.


Non-deep neural network
Deep neural network
Figure 1: Graphical representation of the architecture of an artificial neural network. [15]
Deep neural networks use the hidden layers to reach higher and higher levels of abstraction. The expectation is that a higher number of hidden layers allows for a higher level of abstraction, contributing to an improved performance of the neural network.
Learning in deep networks occurs in an autonomous way, while in traditional machine learning, features are manually extracted and selected in order to create a model able to classify objects.
Studies and researches on neural networks and artificial learning started in the 50s and the subject keeps evolving and improving. The aim of this section is to provide a hint over the history of the development of artificial intelligence, by focusing on its main contributors, from the perceptron model, starting point of the field, through the backpropagation algorithm, where a huge increase in performance was achieved, to the role of vision with the development of convolutional neural networks, which has led to the latest advances in the computer vision field.
The perceptron theory
The perceptron first appeared in a paper published in the late 60s, by the psychologist Rosenblatt [17]. At the time, scientists struggled to create reliable and powerful machine learning algorithms, and the development of Artificial Intelligence was dominated by emulating logical reasoning in machines. The idea of imitating biological computation in artificial neural networks had already been suggested in 1943, by McCulloch and Pitts, but with limited success [16]. Rosenblatt had the goal to study artificial intelligence from a biological point of view, and wanted to focus his work on 2 of the 3 fundamental questions related to the field that had yet to be answered:
-
In what form is information stored, or remembered?
-
How does information contained in storage, or in memory, influence recognition and behavior?
His approach to the second question was against the common idea of the time: it was expected that a certain mapping between stimulus and stored pattern could be made. Therefore, understanding “the wiring” would be the key to the problem.
Rosenblatt developed the uncommon idea that there was no mapping but rather a system of retention of the information in the form of new links being created. This was the birth of the current idea of the perceptron.
The theory of the perceptron had already been proposed by Hebb [65] and Hayek [66], who designed 5 main principles that Rosenblatt would follow in his paper.
-
The wiring is not identical from one organism to another.
-
The wiring is able to change overtime (plasticity).
-
Similar input tends to form connections in a similar way
-
Positive and negative reinforcements can facilitate the formation of on going connections
-
Similarity between systems is seen as the tendency of similar inputs to generate similar outputs.
This is considered to be the birth of the theory of the perceptron. But what did this primitive perceptron look like? It was very close to modern perceptrons. They received binary inputs that were transmitted to what were called associations cells (A-unit) in a ‘projection area’. These cells received a number of connections from inputs, and if the sum of these inputs is greater than a given threshold, the cell fires. These cells are associated in layers and the area between layers is called an association layer. These connections can have a feedback coming from next projections area.

Figure 2: Representation of Rosenblatt’s perceptron structure [18]
Rosenblatt discovered that these feedback allowed the perceptron to learn. By attributing values to cells, that would grow as the cell is being used, one could enhance the impact they had on the global network. This could be done by either freezing the perceptron after forcing it to give the correct answer, by example.
Rosenblatt derived the analytical equations to show that this allowed the perceptron to learn to recognize a class he had already encountered on a learning set. He then tried to test the system to recognize unknown objects of known classes (has the system learned or just memorized the learning set?).
After experiments, he came to the following conclusion:
In the limit it makes no differences whether the perceptron has seen a particular test stimulus before or not; if the stimuli are drawn from a differentiated environment, the performance will be equally good in either case.
Rosenblatt just discovered that neural networks can learn from their data set and apply gained knowledge to new situations.
But Rosenblatt also noticed that the accuracy of the predictions decreases as the number of possible classes rises. If classes are mutually exclusive that is. He therefore introduced the idea of discriminative features: instead of looking to identify objects directly, the network would identify features that would lead to identify the accurate object. For example, if the goal is to recognize common animals, there might be too many classes to cover. So, the network could instead learn to recognize some features: big or small? Hairy or not? Ears or not? 2 or 4 legs? Etc. A small hairy animal on 4 legs could be considered a cat. This is just to give the idea.
In short, Rosenblatt came to the following conclusions:
-
These systems can learn to associate specific responses to specific stimuli
-
In ideal environments, the accuracy diminishes as the number of stimuli learned increases
-
In differentiated environments, accuracy rises as the number of stimuli learned rises.
These conclusions led to the question: what were exactly the limits of the perceptron? And opened the way to the technology.
The backpropagation
In the 80s, artificial learning was one of the main fields of research as it was one of the most unsolved problem of artificial intelligence.
It was discovered that there was no limit to the number of layers in a network and to the connectivity within any given layer. But the main issue remained: large networks took too much time to reach the equilibrium. In order to give an idea, today’s computers can perform billions of operations per second. In the 80s, the order of magnitude was thousands. This means that nowadays computers are one million time faster than the previous ones [20].
After the development of Boltzmann machine learning algorithm [19] and after discovering that it could be utilized to train multilayer networks, there was an explosion of the research in artificial learning and many new learning algorithms were created. The most important is the backpropagation algorithm. The most important contribution to the popularization of the back-propagation for neural networks is an article written by Geoffrey Hinton, David Rumelhart and Ronald Williams appeared in Nature in 1986 [21]. Since then, the article has been cited more than 40,000 times [14].
In the paper the backpropagation method is described: the procedure consists in adjusting the weights of the connections in order to minimize the calculated difference between the present output vector and the desired output vector, by calculating the gradient of the vector function.
Although in the 80s many similar methods were developed, this paper included computer experiments whose results demonstrated the main advantage of backpropagation with respect to other learning methods, namely that it can lead to useful internal representation features. As a result of connection weights adjustments, the hidden units, namely the units that are not part either of the input or of the output, come to represent important features of the task domain.
Two main tasks were studied, that could not be done just connecting the input units to the output ones. The first one is the detection of symmetry.
The second performed task was about storing the information in two isomorphic family trees.
The computer experimentations gave good results, but the strong limitation is that the method is based on the calculation of a gradient of a function, so it is not guaranteed to find a global minimum, it may also be local. However, the experience with many tasks suggests that the algorithm rarely ends up at a local minimum.
It is worth mentioning that the 1986 backpropagation paper cites a paper from the previous year, published on Cognitiva 1985 [21] by Yann LeCun, one of the most important researchers and innovators in deep learning technology.
Yann LeCun presented an independently discovered new method for supervised learning, based on a threshold network structure. The model is composed by input units, output units and hidden units. The process consists on a local iterative scheme minimizing a particular cost function (technical name that is still used in the backpropagation procedures) by modifying interactions between the units. Simulations were performed about the recognition by analyzing the pixels of an image and character recognition. This second method was a major breakthrough in the field when in 1989 LeCun trained a network able to read hand written zip codes on letters, using the Modified National Institute of Standards and Technology database [22].
In 1987, Yann LeCun moved to the university of Toronto, in Canada, to work with Geoffrey Hinton and his research team. Together, they developed the convolutional neural networks, studied in the next section.
LeCun and Hinton, for their studies and researches are two of the most important researchers associated with the development of deep learning.

Figure 3: Graphical representation of the backpropagation algorithm [23]
Convolutional neural networks
Convolutional neural networks (CNNs) play a central role in deep learning technology. Its way of working is inspired by the biological processes which take place in the animal visual cortex animal visual cortex. Most of the tasks which require an image recognition ability, such as medical imaging or autonomous car driving, are performed by such networks.
Although first researches in CNNs took place in the early 80s, one of the most influential publication for the field is the paper “Receptive fields of single neurons in the cat’s striate cortex”, published in 1959 by two neurophysiologists, David Hubel and Torsten Wiesel [24].
By running multiple experiments elaborating the electrical signal from a cat’s brain responding to visual stimula, they demonstrated that specialized neurons in the occipital obe’s visual cortex responds to specific features of an image such as angles, lines, curves and movement.
The two scientists brought to light the functioning of biological visual cortex processes, on which the convolutional neural networks are modelled.
As previously said, the first researches of convolutional neural networks took place in the early 80s: the most important publication is from a Japanese scientist, Fukushima [25]. He developed a network able to recognize patterns which was unaffected by position shifts.
The next major step paper appeared in 1989. Yann LeCun applied the backpropagation learning algorithm to Fukushima’s network, and he released LeNet, namely the first convolutional neural network. He applied his network to hand writing character recognition task [22].
CNNs were not very popular until 2012, when a team from the university of Toronto released the network AlexNet. This network was able to reduce the error rate to a 16%, against the 25-30% of the state-of-the-art models in those years for the image recognition task [26].
These spectacular results launched a huge boost to deep learning. In the recent years, the improvement of convolutional networks approaches has delivered a continuous decrease in the error rates in field of image recognition. Convolutional neural networks are for now the best available technology for computer vision tasks.
The classical architecture of CNNs consists of three different types of layers: convolutional layers, max pooling layers, and fully connected layers.

Figure 4: Structure of a convolutional neural network [27].
Convolutional layer
The convolutional layer is the core building block of a CNN. The layer's parameters consist of a set of learnable filters (or kernels), which have a small receptive field, but extend through the full depth of the input volume.
During the training, each filter is convolved across the width and height of the input volume, computing the dot product between the entries of the filter and the input and producing a 2-dimensional activation map of that filter. As a result, the network learns filters that activate when it detects some specific type of feature at some spatial position in the input.
Max pooling layer
Another important concept of CNNs is pooling, which is a form of non-linear down-sampling. There are several non-linear functions to implement pooling among which max pooling is the most common. It partitions the input image into a set of non-overlapping rectangles and, for each such sub-region, outputs the maximum. An instructive example is shown in Figure 5.
Intuitively, the exact location of a feature is less important than its rough location relative to other features. This is the idea behind the use of pooling in convolutional neural networks.

Figure 5: Example of pooling [28].
Fully connected layer
Finally, after several convolutional and max pooling layers, the high-level reasoning in the neural network is done via fully connected layers.
Discussion
The dream of creating a machine that is able to emulate the biological processes in brain appeared before the invention of the first computer. Today, although technology has made giant steps, this problem is still not solved.
Deep Learning is a most successful example of imitating the brain in visual processing.
In a paper published in 2018 [29], Geoffrey Hinton demonstrates how some variations of backpropagation with a more biological based approach can potentially further improve the performance of backpropagation algorithms.
Section 2.2: From Tic Tac Toe to Alpha*
Games have always occupied a central place in the development of artificial intelligence. Since the beginning of AI, the ability of solving games has served as an objective measure of capacity of a machine to outperform human reasoning.
A central element to artificial game solving is Reinforcement Learning. Reinforcement learning is an area of machine learning concerned with how software agents ought to take actions in an environment to maximize a cumulative reward.

Figure 1: Reinforcement learning scheme
There are multiple Reinforcement Learning algorithms, in this section the most relevant for solving games are explained following the history of the AI applied to games.
How has AI dealt with games through history before Deep Learning?
The first software that managed to master a game was programmed by A. S. Douglas in 1952 on a digital version of the Tic-Tac-Toe game and as part of his doctoral dissertation at Cambridge [31].
This game as well as the Chess or the Go is formally defined as a deterministic, turn-taking, two-player, zero-sum game with perfect information. This means that actions are determined by causes regarded as external to the will, and each player plays only in his turn, and could either win or lose. In addition, they are perfectly informed of all the events that have previously occurred. With such characteristics, any game can be theoretically solved by expanding the game tree and selecting the optimal movement for each position following the Minimax algorithm [32], as it is shown in the Figure 2: Tree expansion for solving Tic Tac Toe. But in the real world, the computation power limitation makes this method infeasible for long games as Chess or Go.
To illustrate this infeasibility, we can compute the number of tree final leaves we will find by expanding the whole Minimax tree, and how long it will take to compute to the best commercial processor (not supercomputer): The most powerful commercial processor launched by intel counts with 28 cores, and a 5Ghz frequency. This means a maximum 28 x 5 x 10⁹ = 140 x 10⁹ operations per second. A full chess game tree has ~35⁸⁰ size. So, in the optimal case where each of the leaf nodes would take only one operation to be computed, it would take 2.39 x 10¹¹² seconds or 7.57 x 10¹⁰⁷ years to compute one full Minimax tree for chess.
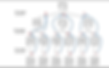
Figure 2: Tree expansion for solving Tic Tac Toe
This approach can be improved reducing the depth of the game by position evaluation. This improvement was the one which led to superhuman performance in chess. It was implemented in the famous Deep Blue machine that defeated then-reigning World Chess Champion Garry Kasparov in a six-game match in 1997 [33]. But this approach, again, faced limitations in solving the game of Go due its larger dimension (chess ~35⁸⁰ vs go ~250¹⁵⁰) [30].

Figure 3: Gary Kasparov vs Deep Blue (1997)
The next step in the development of game solving AI systems was the Monte Carlo Tree Search method [34]. Before Alpha Go, the strongest programs were based on this system. This method first train a policy (or several policies) to predict human expert moves. This policy is used to narrow down the search to a beam of high-probability actions, and to sample actions during rollouts. This approach achieved strong amateur play, but it was still far from the professional performance.
Deep Learning was first used for learning how to solve some classic Atari games, then professional Go level was finally achieved by an AI, and in the most recent past professional level was also achieved in Starcraft II, known for being the most complex strategy game at the moment.
How does Deep Learning implement the solution of games?
Starting in the early 2010s, a research group from DeepMind decided to combine Deep Learning and reinforcement learning techniques with the objective of giving to computers the ability to acquire a certain human-level control to certain domains. This combination got the name of Deep Reinforcement Learning. The first application of Deep Reinforcement Learning by DeepMind was the development of the Deep Q Network agent [35] .
This agent was designed for being able to learn policies from high-dimensional sensory input maximizing some reward function, and it succeeded at solving some classic Atari Games. This agent receiving only the pixels and the game score was capable to make good decisions for maximizing the final reward of the problem.

Figure 4: Deep Q Network solving Atari games
This work opened the possibility for an agent to learn without prior knowledge. At this moment another question was raised: How well could a Deep Learning based agent perform in a complex situation? At this point Alpha Go development began [30].
Also developed in Deepmind labs Alpha Go was able to achieve professional level at Go. This was thought to be at least 10 years far away in time following the classical approaches due its complexity but thanks to the learning ability of the Deep Learning algorithms it was finally solved as explained below.
Alpha Go engine
Alpha Go was trained using a pipeline consisting of several stages of machine learning. It was firstly fed using a 19x19 representation of the board. This board situation was computed using the state-of-the-art convolutional networks which drove to great advances in computer vision in the previous years in systems such as ImageNet [36].
Therefore, from the original Go complexity (breath^depth ~ 25¹⁵⁰ the search depth was reduced by evaluating positions using a value network and the breadth was reduced by sampling actions using a policy network. To that end, the process starts by training the neural network using a pipeline with several stages: (1) supervised learning of policy network, (2) training a fast policy that can rapidly sample actions during rollouts, (3) a reinforcement learning policy network that improves the supervised learning policy network by optimizing the final outcome of self-play games and (4) training a value network that predicts the winner of games played by the RL policy network against itself.

Figure 5: a) Training pipeline, b) description of policy network and value network
An efficient combination of the policy and value networks is achieved with Monte Carlo Tree Search. For training the system these steps were followed:
-
The policy network takes as input the convolutional layers, and as outputs, the probability distribution over all legal moves in order to predict expert moves. The supervised learning policy network is formed by 13 layers and it is trained from 30 million positions. The results showed that it predicted expert moves with 57.0% of accuracy. In addition, large improvements in playing strength were achieved by small improvements in accuracy.
-
The reinforcement learning neural network has the same structure as the previous Supervised Learning policy network with its weights initialized to the same values. These values are updated at each time step t by stochastic gradient ascent in the direction that maximizes the terminal reward (+1 for winning, -1 for losing) at the end of the game. After the evaluation, the reinforcement learning policy was shown to win more than 80% of games against SL policy. They also played against the strongest Go program at that moment, Pachi, getting that the RL policy network won 85% of games, whereas the SL policy network won only 11%. With this reinforcement learning, the policy’s main objective changes from maximizing the accuracy to optimizing the goal of winning the game.
-
The final stage of the training pipeline focuses on position evaluation, estimating a value function that predicts the outcome from position of games played by using policy for both players. This neural network has a similar architecture to the policy network, but it outputs a single prediction instead of a probability distribution. The naive approach of predicting game outcomes from data consisting of complete games leads to overfitting. To mitigate this problem, a new self-play data set was sampled with 30 million distinct positions, each sampled from a separate game. Each game was played between the RL policy network and itself until the game terminated. Training on this data set led to mean squared errors of 0.226 and 0.234 on the training and test set respectively, indicating minimal overfitting.
Finally, AlphaGo combines the policy and value networks in a Monte Carlo Tree Search algorithm that selects actions by lookahead search:
-
Each edge (s, a) of the search tree stores an action value Q, visit count N, and a prior probability P. At each time step t of each simulation, an action between all possible is selected maximizing the action value Q plus a bonus proportional to the prior probability. The tree is traversed following the different max actions.
-
When the traversal reaches a leaf node at step L, the leaf node may be expanded. The leaf position is processed just once by the SL policy network. The output probabilities are stored as prior probabilities P for each legal action.
-
The leaf nodes are evaluated in two different ways, first by the value network, and second by the fast policy, and both outcomes are combined giving a node evaluation V.
-
At the end of simulation, the action values Q and visit counts N of all traversed edges are updated. Each edge accumulates the visit count and mean evaluation of all simulations passing through that edge. Once the search is complete, the algorithm chooses the most visited move from the root position.

Figure 6: Movement selection
Which results were brought by the Deep Learning approach in Go?
To evaluate AlphaGo, an internal tournament was run among variants of AlphaGo and several other Go programs, including the strongest commercial programs Crazy Stone and Zen, and the strongest open source programs Pachi and Fuego. All programs were allowed 5s of computation time per move. The results of the tournament suggest that AlphaGo is many dan ranks stronger than any previous Go program, winning 494 out of 495 games (99.8%) against other Go programs.
Finally, the distributed version of AlphaGo was evaluated against Fan Hui, a professional 2 dan, ending with AlphaGo winning 5 games to 0. This is the first time that a computer Go program has defeated a human professional player, without handicap, in the full game of Go.
With this AlphaGo reached a professional level in Go, providing hope that human-level performance can now be achieved in other seemingly intractable artificial intelligence domains. It is also important to observe that it was done by training directly from gameplay purely through general-purpose supervised and reinforcement learning methods. The AlphaGo victory is a formidable step forward in the development of machine learning and artificial intelligence.
What is next?
After the AlphaGo development, Deepmind also launched an ambitious project based on the famous real time strategy game called Starcraft II. During one year the team worked in collaboration with Blizzard, the developer of Starcraft II game, to set up an API that gives the possibility for external tools to interact with the game [38].
Once the API was functional the development of an agent based to master the Starcraft II game started. Starcraft II is one of the most complex strategy games existence. Unlike all classical board games, Starcraft II is not turn taking, there is no perfect information and the board is not fixed.
Finally, early this year 2019, the team published videos and articles showing the new agent Alpha* beating top Starcraft II players [37]. Those results are once again impressive, taking into account the complexity to master such a game to master even for humans.

Figure 7: Alpha* in game situation
With that new step reached, and despite of its limitations (the project is still ongoing) the team is demonstrating potential of deep learning to some of the most complex reasoning tasks our world. Nowadays a great number of researches are also looking for the possibility to extend the application of this Deep Learning methodology to other application domains such as management of power system, healthcare diagnosis, recommender systems, etc.
Section 3.1: Society 2.0
The Society of the future
The impact of deep learning in varied domains of our life is remarkably wide-ranging and expanding. This is what we call the Society 2.0, where big data is essential. Fields such as transport, medicine or energy will be strongly impacted by deep learning and its applications.
The most immediate revolution could come from transportation, with the widespread development of autonomous vehicles.
On the other hand, deep learning is developing at a fast pace in medicine, where imaging is fundamental to diagnosis. It could contribute to accelerate the development of drugs for pathologies such as Alzheimer or cancer, whose healing means are unknown.
Finally, deep learning could help reduce environmental issues by improving the efficiency of human operation in large facilities. A particular application could be the operation of energy devices and the integration of renewable energies in the electric grid. Hence, this revolution also expected to mitigate some problems as relevant as the climate change or the emission of greenhouse gases.
Transportation 2.0
One of the most immediate applications of Deep Learning is the self-driving or autonomous cars. These vehicles are starting to become a reality, and the first autopilot systems are starting to be commercialized. One of the most famous is Tesla autopilot [40].
Since the 1990s, a time when autonomous driving was still in science fiction books or movies, engineers and technicians have been working on driver assistance systems. In the coming decade, the automotive industry will change more dramatically than in the last 30 years.
These applications will guarantee an extraordinary reconstruction of the transport system. It will also have consequences on the operating of trains, planes, buses and ships. A new era of highly automated driving will increase the flexibility of the schedule of public transport and its versatility will offers new solutions to traffic jams or traffic accidents.
The autonomous car example:
The cutting edge in this field is the autonomous car. The architecture of its technical operation is illustrated in the figure below. It describes how the information taken from the different sensors is used as an input by the neural network which finally decides the best possible action.

Figure 1: Autonomous car architecture
In the recent years, the most dramatic advances in autonomous driving have come from the vision based. Autonomous cars use a camera as their main sensor. Nowadays, there are two major paradigms for vision-based autonomous driving systems: mediated perception approaches that parse an entire scene to make a driving decision, and behaviour reflex approaches that directly map an input image to a driving action by a regressor.
The mediated perception approaches try to build a consistent representation of the car close surroundings. They achieve this goal by using multiple sensors as shown in the Figure 1 for recognizing driving relevant objects. The decision making based on this visual information is processed by a deep neural net.
On the other hand, the behaviour reflexes approaches construct a direct mapping from the sensory input to a driving action. To learn the model, a human drive the car along the road while the system records the images and steering angles as the training data. Although this idea is very elegant, it can struggle to deal with traffic and complicated driving situations.
Both approaches have limitations. The main problem of mediated perception approaches is its huge complexity, since it considers all the objects in their surroundings while only a few of them are relevant for the driving task. On the other hand, the main drawback of the behaviour reflexes approaches is the low level of abstraction that fail in understanding the real driving situation, since for similar inputs different actions should be taken. A possible neat solution consists in only trying to find a representation that directly predicts the affordance of driving actions. This approach is called Direct Perception Approach.
The main process of the development in autonomous cars can be summarised in three different steps: first, an extended collection of data is required; secondly, it starts the training of the model with the mapping from an image to affordance; and finally the training will conclude with the mapping from affordance to action. The model will predict some indicators (speed, relative position to lane markings, heading angle, distance to the preceding cars, etc), process them and send back the proper command to the engine and its management.
The main goal is that the host car can achieve stable and smooth car-following under a wide range of speeds and even make a full stop if necessary. Nevertheless, more resources must be trained in order to achieve a better performance which can be deployed massively as a real and safe autonomous car.
Nowadays, partly automated driving cars are already a reality and they are commercialized with systems that take over tasks and responsibilities from the human driver. Some examples of these systems that make daily driving much easier include the remote-controlled parking function, the automatic brakes, the steering and lane control assistant, the traffic jam assistant, and the speed control.

Figure 2: Autonomous car
Medecine 2.0
Since the 1970s, when it was possible to scan medical images in a computer for the first time, researchers started to create systems for automated analysis (Lijtsiens). In modern healthcare, the importance of digital medical imaging has widely increased, hence it has become an indispensable role in clinical therapy.
The most applied methodology of deep learning practiced in medical imaging is the Convolutional Neural Networks (CNN). As it has been explained in depth in the section ‘2.1. Intelligent people who did intelligent machines’, CNN way of working is inspired by the biological processes which involve the connectivity pattern between animal visual cortex and neurons.

Figure 3: Structure of a convolutional neural network
The main application in medical image analysis is the image classification. Nowadays, database can deal with more than 100.000 images for more than 2.000 different diseases. Au automated classification could be very useful to radiologists. Beyond image classification, there are raising expectations to use deep learning in medical diagnosis. Some people envision the diagnostic of many diseases from a simple picture taken with smartphone.
On the other hand, medical imaging will be aided by other methodologies, which can improve their accuracy or their scope. Independent Component Analysis (ICA) could be a concrete example which will complement these new techniques in complicated and enmeshed fields as brain diseases.
In a recent research of 2017 [45] a CNN has been trained in order to diagnose the most common deadliest skin cancers. The algorithm of this investigation has achieved a performance level which is comparable to the human performance of 21 dermatologists.
This information could lead to think that one day there will be no need of doctors. So far, however, deep learning has only started to complement doctor opinions and enhance their accuracy. The likely reality is that a supervision from experts will be always needed.
Furthermore, in cases such as cancer and other problems, which require a very early diagnosis to be healed, deep learning could help to detect diseases at a very beginning phase.

Figure 4: Magnetic Resonance Imaging (MRI)
Control and automation
The complexity of some environments may become a big headache for the operation of complex systems, where there are innumerable constraints with conditional restrictions or with dynamic elements.
Deep learning will enhance several processes and improve dramatically their data efficiency or their task performance. Moreover, it will produce vastly superior results than standard methods. These kind of algorithms are specialized for training and evaluation of continual learning methods, as well as general reinforcement learning problems. Sophisticated techniques with innovative approaches can come to know how to navigate from raw sensory input, approaching human-level performance even under situations where the control objective alters frequently.
The energy Market example:
The operation of systems related to energy markets provides a relevant illustration. With the rapid development of renewable energy infrastructures, volatility or unpredictable generation will increasingly require the support of more complex algorithms. Deep learning is also raising expectations for an improvement of energy market efficiency.
Under most circumstances, the variability and the unpredictability of energy output of renewable energy technologies will involve an impediment to dealing with their integration into the energy supply network and may invoke additional system costs, especially when they will reach greater shares [41]. In this connection, growing the share of clean sources in the energy mix will demand policies to encourage re-modelling in the energy network.
Some alternatives to decrease these risks include the development of complementary schemes with a flexible operation in generation or energy storage technologies [42]. In this manner, the deployment of dispatchable hydropower technology can play an important function in view of the fact that this technology pumps water uphill into reservoirs during periods of low demand, to be released for generation while appears peaks electricity demand. In addition to delivering energy, the fast ramping characteristics of hydro units makes them suitable for delivery of various balancing products that are needed in order to maintain the security status of the system.

Figure 5: Hydroelectric station
The operator of a hydroelectric power station has to cope with the dilemma of adding an offer to buy energy, incorporating a bid to sell energy or doing nothing in order to maintain their resources. This delicate decision is called the identification of the opportunity cost of trading. Additionally, the determination must be taken every minute in a continuous intra-day market, consequently the optimal solution, which maximize the cumulative profits earned over the entire trading horizon, is significantly more laborious. Besides, the decision making process also involves long-term decisions: the generation company must decide how much capacity offers to the energy market and how much offers to the balancing markets.
In recent years, several studies have been published in order to solve this complex problem faced by the operators of storage devices. Some of them are based on deep reinforcement learning and they usually are modelled as Partially Observable Markov Decision Process [44].
The optimal choice for each moment is composed of two different high-level actions. During the first one, defined as ‘Idling’, no transactions are effectuated and the system aims at identifying the profits that the storage device could get over the rest of the trading horizon. Afterwards, the second action, defined as ‘Optimizing based on current knowledge’, trades by the requested orders and the situation of the storage device. This latter step takes into account the technical limitations of storage devices. The trading agent learns the optimal policy which provides higher revenues than traditional methods.
Deep learning will take more importance in disparate sectors, because of the rapid growth of databases. According to energy markets, this growth will be promoted by the use of shorter time-slots, the optimization of members’ portfolios closer to real-time and the decrease of imbalance costs. The creation of new interconnections between disparate systems will further increase the amount of data that operators will have to analyse.
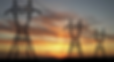
Figure 6: High Voltage Transmission Line
Section 3.2: Deep Learning, for the better and the worse
Introduction
Deep learning is a recent technology that raises lots of expectations. But this technology eminently relies on data, and data is not a resource that anybody can access in unlimited quantity [46].
Individuals only have access to their personal data, or to the data that are available on the internet. Instead companies can more easily harvest data through their products, websites, applications. Users of these services provide data that the company can collect [47].
This leads us to a first grand challenge. The harvesting of data can lead to a number of issues concerning privacy and morality, the dilemma being further amplified as harvesting data becomes more profitable.
The new technologies also bring a social dilemma: they will outperform human performance in efficiency and speed, leading to higher profits for the producer but potentially putting millions out of jobs.
This last section aims at highlighting some of the societal and ethical issues raised by the development of deep learning.

Figure 1: Will robots put humans out of jobs?
The impact on jobs
The world has come a long way technology wise. We have come through many technological revolutions and some had such an impact that they are considered as a global revolution. These revolutions always revolve around the same concept: new technologies replace humans activities by outperforming them. In this aspect, Deep Learning isn’t any different. The fears arising from technological revolutions are often similar too, and one of them is the loss of jobs and need for humans, that would be over classed by new coming technologies. This fear is back in our society, as illustrated by many recent publications in the media :[48,49,50,51]
In the past, this fear often proved to be excessive, many countries have reached full employment after the three last major technological revolutions. These revolutions were, in order: the first industrial revolution (+-1765), with mass extraction of coal/steam engines/metal forging processes, the second industrial revolution (1870), with gas, oil and electricity, chemical synthesis. Arrival of large factories (Ford). The third industrial revolution (1969) came with nuclear energy, rise of electronics, and arrival of computers and telecommunications, and bio-technologies.

Figure 2: Industrial revolutions
These revolutions profoundly changed the way humans interacted with their environment and changed the society, and could momentarily put people out of jobs, but new jobs always appeared and replaced the old ones. So will it be the same this time? This is what some experts tend to agree upon: even if the question is still broadly open [52]. An evolution of the skills required for labor forces could be the answer, or a shift toward more jobs focused on social skills or creativity. A new technology can be the source of big profit, which is potentially money to be reinvested in other sectors. For example, more teachers could be hired and better paid or better trained, bringing the opportunity to have improved education overall. We also often hear that our medical infrastructures are under staffed and underpaid( UK : [53]) (US : [54]) (Europe in general : [55]). If doctors were to be assisted by AI, this could potentially reduce the problem of waiting lines in hospitals. In remote locations where doctors are lacking, a simple computer in an office could dispense diagnosis and solve the health problem for the inhabitants. If less doctors were needed, the sparing of their salaries could be used to hire more nurses.
These 2 examples show how jobs can simply be shifted or used in places where they are most needed. In the end, it is hard to say if the balance will be a loss or a gain. The following publication provides a more complete discussion on the subject: [56]
Potential impact on tomorrow society
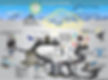
Figure 3: AI in our everyday lives
What will DL potentially be able to bring us as technologies?
The previous section has illustrated some of the potential technological impacts of Deep Learning. One of the most noticeable current effect is the medical field innovation [57]. Deep Learning is becoming effective at identifying diseases and is expected to soon outperform human expertise. We can easily see a future where diseases will be treated more efficiently and discovered faster in individuals. Self-driving cars will also improve safety during travels and passively save lives. Many experts believe that people will no longer own a car int the future [58]. This evolution would drastically reduce the need for cars, and the need for parking. Other sectors potentially impacted by deep learning include education, law, and research. Some even imagine that deep learning could help to explore space.
Another field that could be vastly improved thanks to deep learning is security. Fiscal fraud can be detected, spams can be recognized, cameras using face recognition can track criminals in the streets.
This leads to a trade-off though. Security has always been something that could easily come at the price of liberty. If a camera is trained to recognize a criminal’s face, it will have to look at everybody’s face and analyze it in the process. In China, all the population is or will soon be watched by cameras able to recognize every individual [59].The same technology that is used to detect SPAMS can be used to detect other kind of content. This begs the question of the limit that we should put on where to use these technologies[60,61].
General ethics considerations

Figure 4: What should be the limits ?
Ethic is a recurring theme in Deep Learning. Two ethical questionsdirectly come to mind : is it ethical to collect more data if collecting more data means improving the performance of an algorithm but also reduces individual privacy ? Are the optimal decisions of an artificial network necessarily ethical ?
A common example is the trolley problem. In this thought experiment, a train is going wild on a track and 5 people are tied to the trails, and are going to die. The driver can decide to pull a switch and redirect the train to another track, where a single person is tied. The moral dilemma comes from actively killing someone for the purpose of saving 5 others.
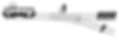
Figure 5: Trolley problem
Such scenarios are idealised but illustrate potential issues of artificial decision making. An autonomous car might have to take a decision in the case of broken brakes. Should it decide to throw the car out of the road, potentially killing the passengers but mitigating the risk of killing others ?
Another example is in insurance. Deep learning has effectively been used in some USA insurance companies to compute the price clients would have to pay for their insurance [62]. The cost function that is optimised in training the artificial network is not necessarily ethical. So far, many states decided to forbid the technique.
This bias can be seen in some experiments. Some years ago, an AI was launched on Twitter by Microsoft, in the hope that people talking to it would make it smarter and smarter. The app had to pulled off after one day, because the AI became racist and totally inappropriate. The bias induced by ill motivated users had a strong influence. Is it ethical to train a decision-making system with potentially biased data ? [63]
Deep learning can also be used to make weapons more efficient. This is also a debated issue, and many scientists believe that AI in general, including Deep Learning, should not be used in a military context.
The other important ethical issue is the gathering of data. To gather more data, many companies add spying devices in their products. Reported examples include Chinese hardware producers that have installed mini OS in their processors to send data on what is being processed, or Facebook that keeps tracks of all discussions, photos, posts, of any customer, even if deleted from the website, to such an extent that they can have profiles build from people that are not even registered on Facebook through mentions from their relatives on the website. To date, most users are largely unaware of their private data being collected. Is it ethical to take personal data from an unaware teens? To register private discussions and photos? To listen to peoples discussions at any time through smart devices? And to infer data from it concerning people that did not even register in the first place? [64]
Conclusion
Deep learning is a technology, and one that has the potential to be use in many applications. And as any other technology of similar importance, its usage can be beneficial or detrimental to human kind. It is hard to tell in advance what the impact of such technologies will be, as history has shown, and what will be the consequences for us. But it is certainly a responsability of the society to mitigate the detrimental consequences of a new technology.
Conclusion
Deep learning is a recent development of machine learning that has had already a great impact on society. It has allowed humans to create numerous new devices and to solve challenging problems in multiple fields: medicine, autonomous cars, AI for video games, energy sector. Yet it is far from being the only interesting face of this technology: its history shows us how long it can take for a promising technology to become popular and efficient, and how the original idea of it can be far away from its end use. History informs us about the complexity needed to achieve such results in specific tasks and how correlated it was with the early predictions and deductions of scientists like Rosenblatt. We have used this historical insight to examine its possible impact on our future, and how it could shape society for the better or the worse. The last section included an analysis of societal and ethical issues raised by the development of Deep Learning. Much of the above analysis suggests that Deep Learning is indeed a technological revolution, even if history teaches us that revolutions are sometimes short-lived when seen from distance.